
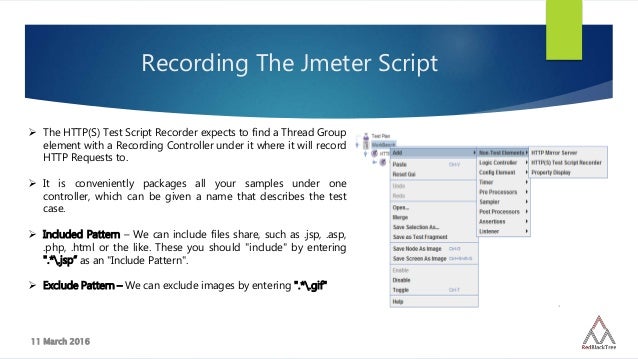
Navigate to the /perftools/deployment/loganalytics directory.ĭeploy the logAnalyticsDeploy.json Azure Resource Manager template. To deploy the Azure Log Analytics workspace, follow these steps: Once you've successfully deployed this library to an Azure Databricks cluster, you can further deploy a set of Grafana dashboards that you can deploy as part of your production environment.Ĭonfigure your Azure Databricks cluster to use the monitoring library, as described in the GitHub readme. This library enables logging of Azure Databricks service metrics as well as Apache Spark structure streaming query event metrics. Azure Databricks does not natively support sending log data to Azure monitor, but a library for this functionality is available in GitHub. In Azure, the best solution for managing log data is Azure Monitor. The first step is to gather metrics into a workspace for analysis. Monitoring is a critical component of operating Azure Databricks workloads in production.
#APACHE JMETER PERFORMANCE METRICS HOW TO#
This article shows how to set up a Grafana dashboard to monitor Azure Databricks jobs for performance issues.Īzure Databricks is a fast, powerful, and collaborative Apache Spark–based analytics service that makes it easy to rapidly develop and deploy big data analytics and artificial intelligence (AI) solutions. The work required to update the spark-monitoring library to support Azure Databricks 11.0 (Spark 3.3.0) and newer is not currently planned. Azure Databricks 11.0 includes breaking changes to the logging systems that the spark-monitoring library integrates with.
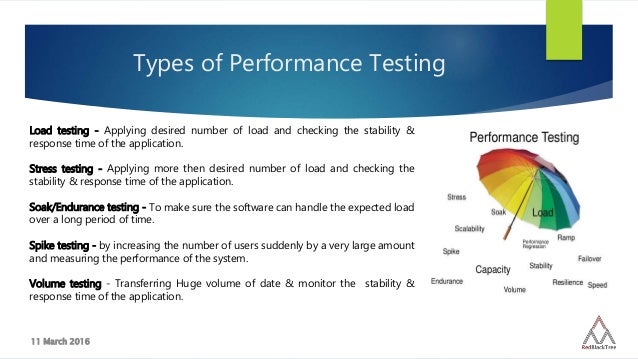
The library supports Azure Databricks 10.x (Spark 3.2.x) and earlier. This article relies on an open source library hosted on GitHub at.
